Data Enhancement vs Data Enrichment: The Differences
In today’s data-driven world, businesses rely heavily on information to make informed decisions and gain a competitive edge. Two terms often used interchangeably are data enhancement and data enrichment. Despite their seeming similarity, they possess distinctive attributes that cater to different aspects of data management and utilization.
Understanding Data Enhancement and Data Enrichment
Data lies at the core of any organization’s operations, offering insights into customer behavior, market trends, and operational efficiency. Both data enhancement and data enrichment contribute to improving the quality and utility of data, but they do so in distinct ways.
Data Enhancement: Deepening the Insight
Data enhancement involves refining existing datasets by adding supplementary information that provides deeper insights. This process involves appending data with relevant details that were previously missing. For instance, consider a retail business that holds customer information such as names and addresses. By enhancing this data, the business could add demographic details, purchase history, and social media profiles, thus creating a more comprehensive customer profile.
Data Enrichment: Expanding the Horizons
On the other hand, data enrichment is about expanding the dataset by incorporating external information from reliable sources. This can involve filling in gaps in existing data or adding entirely new dimensions. For example, a company specializing in real estate might enrich its property listings by including neighborhood crime statistics, nearby schools, and public transport availability.
The Role of Data Enhancement and Data Enrichment in Business Growth
Both data enhancement and data enrichment play pivotal roles in facilitating business growth and decision-making.
Data Enhancement’s Business Impact
Data enhancement leads to a richer understanding of customers and their preferences. By adding layers of information, businesses can tailor their marketing strategies more effectively. For instance, an online retailer can identify high-value customers and customize promotions based on their past behavior and preferences.
Data Enrichment’s Business Impetus
Data enrichment broadens a business’s perspective by incorporating external context. For instance, a financial institution could enrich its customer profiles with economic indicators to provide clients with personalized investment advice aligned with market trends.
Data Enhancement vs Data Enrichment
Let’s take a closer look at the differences between data enhancement and data enrichment through a comparison table.
Aspect | Data Enhancement | Data Enrichment |
---|---|---|
Goal | Deepen existing insights | Expand dataset with external data |
Method | Add supplementary details | Incorporate data from reliable sources |
Use Case | Personalization, targeting | Market analysis, strategic planning |
Example | Enhancing customer profiles | Enriching property listings |
Understanding the Implementation Process
Implementing Data Enhancement
The process of data enhancement involves several steps:
- Data Assessment: Evaluate existing data for gaps and inaccuracies;
- Data Appending: Acquire relevant data from credible sources;
- Data Integration: Merge appended data with existing records;
- Quality Assurance: Verify the accuracy and consistency of the enhanced dataset.
Implementing Data Enrichment
The data enrichment process follows these stages:
- Data Analysis: Identify gaps and potential sources for enrichment;
- External Data Collection: Gather supplementary information from reliable sources;
- Data Integration: Integrate external data with existing records;
- Data Validation: Ensure the credibility and relevance of the enriched data.
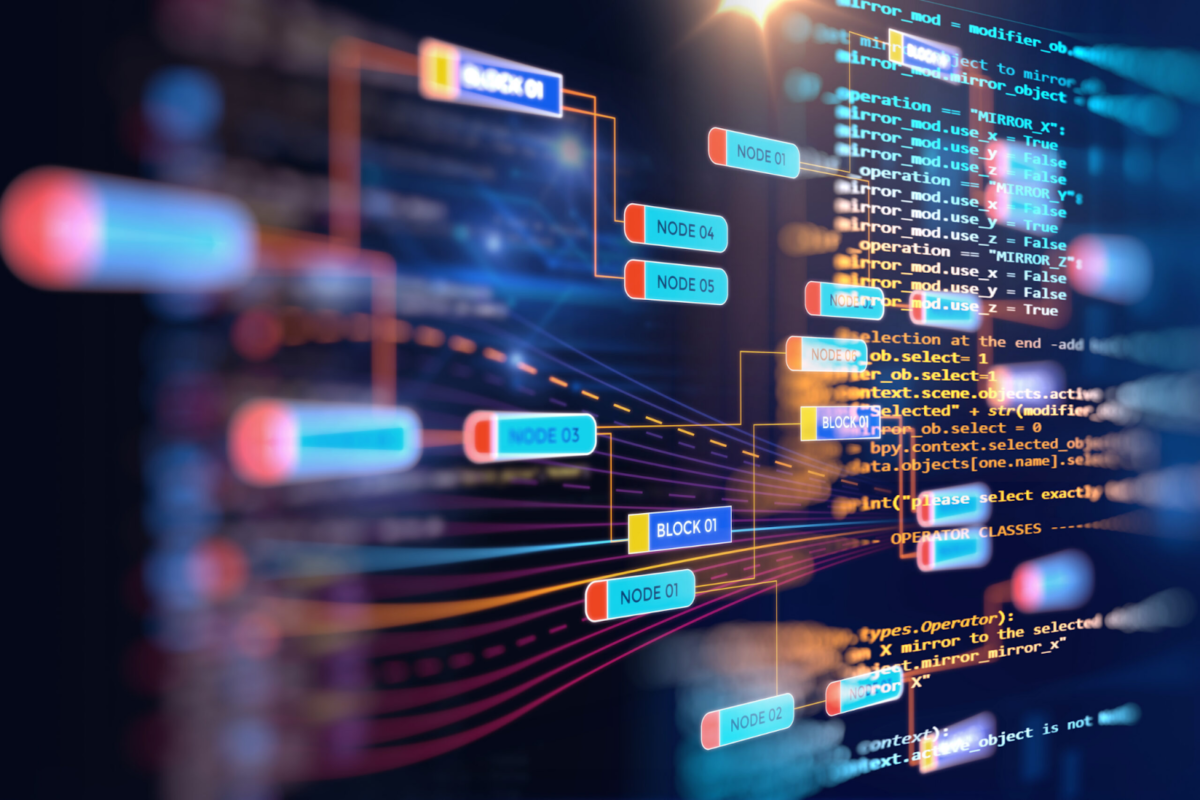
Data Enhancement vs Data Enrichment: A Practical Scenario
To grasp the distinction better, let’s consider a scenario involving a marketing campaign.
Imagine an e-commerce company planning to launch a promotional campaign. By leveraging data enhancement, the company could analyze past purchases, customer behavior, and preferences. This information allows them to personalize the campaign’s content and offerings, increasing the likelihood of engagement and conversions.
Now, let’s add data enrichment to the mix. The e-commerce company could enrich its customer data with external data sources such as social media activity, online reviews, and competitor analysis. This enriched dataset provides a more comprehensive view of customer sentiment and market trends, enabling the company to tailor its campaign to current market sentiments.
Key Considerations for Choosing Between Data Enhancement and Data Enrichment
When deciding between data enhancement and data enrichment, several factors come into play. Each approach has its merits, and understanding these considerations can help businesses make informed choices.
Nature of Business Goals
Consider your business objectives. If your aim is to gain deeper insights into your existing customer base and refine your marketing strategies, data enhancement might be the ideal choice. On the other hand, if you’re looking to expand your market reach and gain a broader perspective on market trends, data enrichment could be more beneficial.
Data Quality and Coverage
Evaluate the quality of your existing data. If you have comprehensive data but lack certain dimensions, data enhancement might suffice. However, if your data is limited in scope, data enrichment can provide you with a wider pool of information to work with, enhancing the accuracy of your analyses and decisions.
Resources and Expertise
Consider the resources and expertise available within your organization. Data enhancement may require integrating additional data points into your existing datasets, which demands database management skills. On the other hand, data enrichment involves sourcing external data from reliable providers and integrating it effectively.
Ethical and Legal Considerations
Both data enhancement and data enrichment involve handling customer data. Ensure that your data practices adhere to legal regulations such as GDPR or CCPA. Data enrichment, especially with external data sources, might raise additional privacy concerns, so implementing stringent data protection measures is crucial.
Real-world Examples: Data Enhancement and Data Enrichment in Action
Example 1: Data Enhancement in Healthcare
Imagine a healthcare provider seeking to improve patient care through data utilization. By enhancing patient records with additional medical history, diagnostic reports, and lifestyle data, healthcare professionals can offer personalized treatment plans, reducing the risk of misdiagnosis and enhancing patient outcomes.
Example 2: Data Enrichment in Financial Services
In the financial sector, a credit scoring agency could leverage data enrichment to enhance credit risk assessment. By incorporating economic indicators, employment trends, and market forecasts, the agency can provide a more comprehensive evaluation of creditworthiness, assisting lenders in making more informed lending decisions.
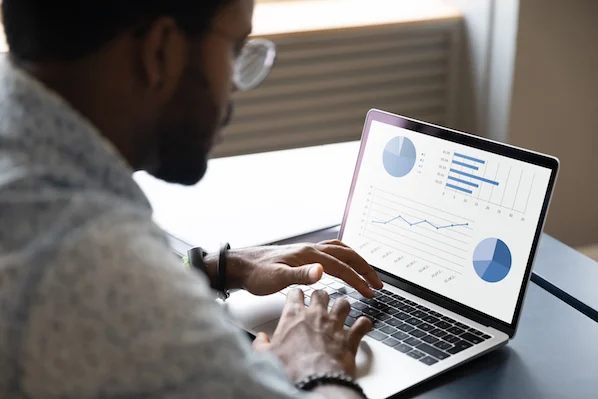
Exploring Hybrid Approaches: The Synergy of Enhancement and Enrichment
While data enhancement and data enrichment have their distinct advantages, a hybrid approach that combines both strategies can yield even more robust results. By first enhancing existing data and then enriching it with external context, businesses can leverage the strengths of both approaches for a more comprehensive understanding of their target markets and customers.
Implementing Data Enhancement and Data Enrichment: Best Practices
Successfully implementing data enhancement and data enrichment requires a strategic approach. By following best practices, businesses can maximize the benefits derived from these processes.
Data Source Evaluation
When choosing data sources for enhancement or enrichment, prioritize reliability and relevance. Ensure that the external data originates from reputable sources that align with your industry or market segment. Verify the accuracy of the data to prevent any potential negative impact on decision-making.
Data Integration and Cleansing
Effective integration of enhanced or enriched data into your existing datasets is crucial. Employ data cleansing techniques to eliminate inaccuracies and inconsistencies. This ensures that the added information harmoniously coexists with your current data, resulting in accurate and actionable insights.
Continuous Monitoring and Updates
Data ages and evolves, making continuous monitoring and updates essential. Set up processes to regularly review and refresh your enhanced or enriched datasets. By staying up-to-date, you ensure that your insights remain relevant and reflective of the current landscape.
Privacy and Security Compliance
Given the sensitivity of data, prioritize privacy and security. Comply with relevant data protection regulations and obtain necessary consents when working with customer data. Implement robust security measures to safeguard both existing and enhanced/enriched datasets from unauthorized access.
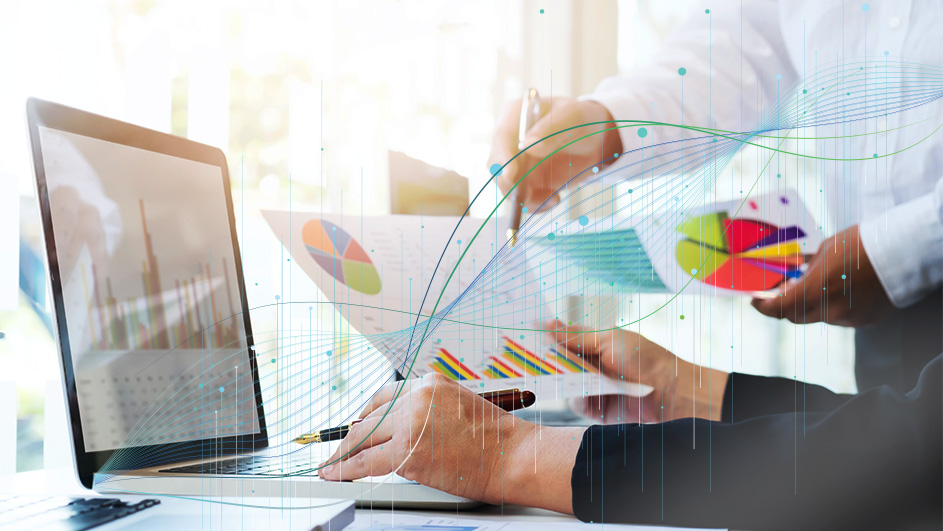
Navigating Challenges and Overcoming Pitfalls
While data enhancement and data enrichment offer significant advantages, they are not without challenges. Understanding these challenges and having strategies to overcome them is vital for successful implementation.
Data Accuracy and Consistency
External data used for enrichment might not always be accurate. Establish validation procedures to verify the credibility of external sources before integrating them. Additionally, ensure that the appended or enriched data aligns with your existing dataset’s structure and quality standards.
Ethical Considerations
Balancing data utilization with ethical considerations is paramount. Transparently communicate to customers how their data will be used and seek their consent whenever required. Strive for a balance between utilizing data for insights and respecting individuals’ privacy.
Resource Allocation
Both data enhancement and data enrichment demand resources, including time, personnel, and technological infrastructure. Evaluate your organization’s capacity to implement these processes effectively and allocate resources accordingly.
Managing Complexity
As datasets grow in complexity, managing enhanced or enriched data can become challenging. Implement efficient data management practices, such as data cataloging and data lineage tracking, to ensure clarity and accessibility.
Concluding Thoughts
In the realm of data management, both data enhancement and data enrichment serve as crucial tools for businesses seeking a competitive advantage. While data enhancement refines existing insights, data enrichment broadens horizons with external context. By understanding the nuances of these processes, businesses can harness the power of data to make informed decisions and drive growth.
Leave a Reply